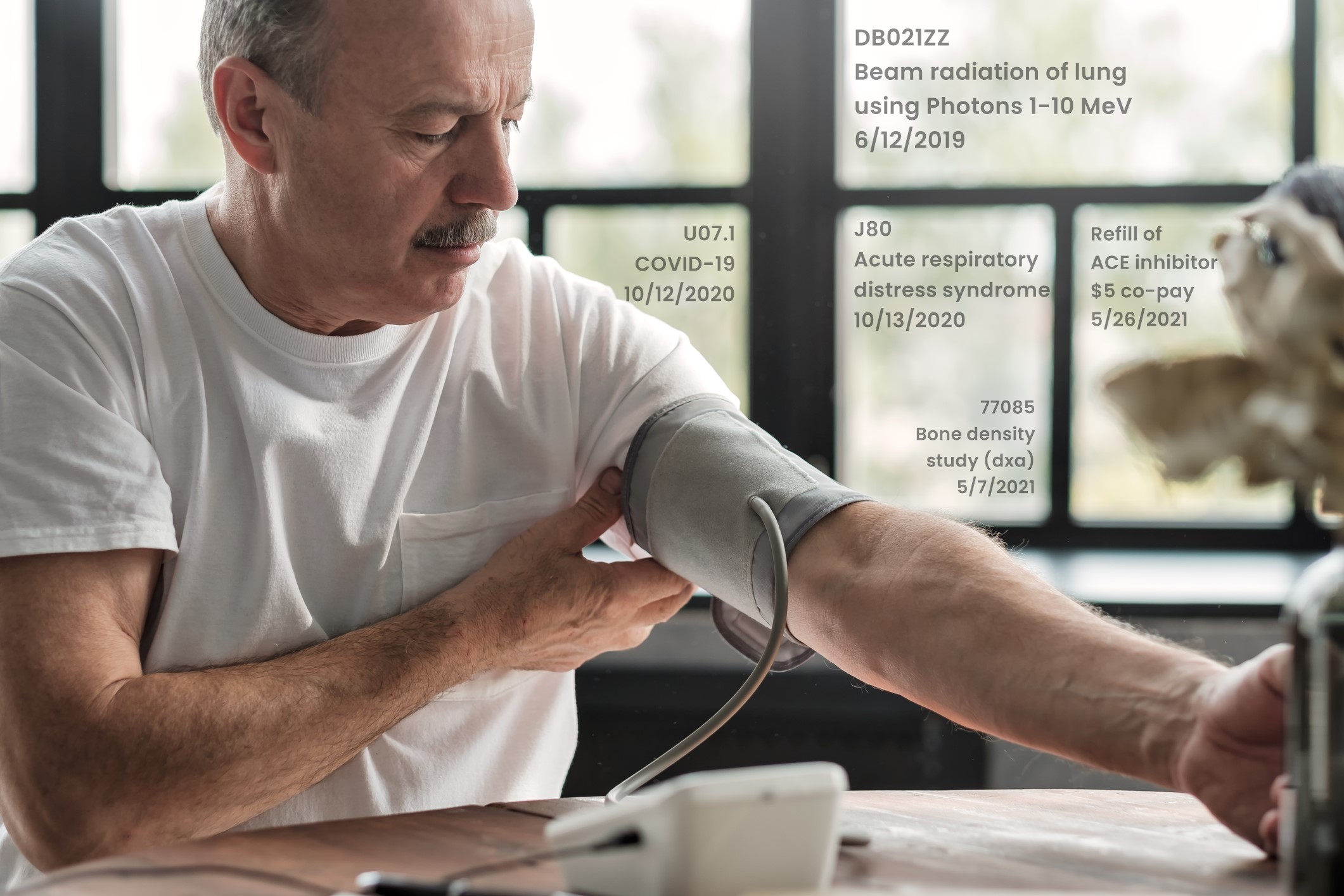
Mateo is a 59-year-old travel photographer who moved from Philadelphia, Pennsylvania to Phoenix, Arizona in October of 2019. Mateo sees his primary care physician (PCP) at a nearby medical center, who’s helping him manage his high blood pressure with ACE inhibitor medication. Before moving to the Valley of the Sun, Mateo underwent radiation treatment for non-small cell lung cancer, which is now in remission. In October of 2020, he contracted COVID-19. Mateo spent four days as an inpatient for acute respiratory distress (ARDS). His hospitalist discharged him with mild symptoms, which resolved at home. In January of 2021, his PCP administered his COVID vaccination. An avid cyclist, Mateo recently mentioned some minor hip pain following a spill on his ten-speed. His PCP ordered a DEXA bone density test, which showed no fracture but did contain some light spots indicative of osteopenia.
Stream our educational webinar,
Revealing the Patient Journey with Linked Claims and EHR
Clinical researchers could learn a lot from Mateo. Analyses of his full medical record, combined with prospective monitoring, could generate insights in areas from health economics to drug safety. Examples include:
- Medication adherence, persistence, and treatment cost
- Inpatient treatment protocols for COVID-19
- Immune response to vaccination in cancer patients previously treated with radiation therapy
- Risk of fracture among osteoporotic patients
Given all the insights researchers could discover, where should they start looking? Two real-world data sources top the list of candidates: EMR data and claims data.
Electronic medical records (EMR) contain a wealth of timely information that spans demographics, diagnoses, encounters, laboratory results, procedures, and medications. Captured in the care setting, EMR data charts the course of a patient’s disease at a level of detail few other sources can provide. However, the data doesn’t reflect activity outside of the care setting, such as prescription fills. The data is siloed, too. For a patient who receives care at more than one hospital, any one EMR record will be incomplete.
Closed claims data, on the other hand, tells a continuous story. Sourced directly from payers, claims data captures care across providers and includes prescription drug transactions. The story is rich in plot, covering all diagnoses, procedures, and prescriptions. But it’s short in some key areas, namely clinical. Lab values, for example, aren’t reported in claims.
EMR vs. claims data. Which should you use for your next analysis? Depending on the question you need to answer, relying on both sources linked together may be your best choice. In many cases, it’s your only choice.
Consider Mateo’s inpatient stay after contracting COVID-19. EMR data for that encounter will provide an admission and discharge dates, along with related diagnoses like Mateo’s ARDS. If integrated with lab databases, EMR will even supply critical measures like viral load and blood chemistry. Only closed claims, on the other hand, will also supply a cost for each drug and service.
Linked together, EMR and claims data on Mateo’s inpatient stay make possible a host of analyses that would be impossible based either source alone. Without both sources, HEOR researchers simply could not investigate the relationship between clinical characteristics upon admission, taken from EMR, and the cost of inpatient care, taken from claims. That relationship is much more than an academic question, with COVID continuing to fracture lives and economies.
If a linked record can extract so much intelligence from a single inpatient visit, what else might it do? Claims data is the one source that reflects both Mateo’s cancer care in Pennsylvania and his preventative care in Arizona. Without that source, a researcher investigating post-injection events, like re-infection with SARS-CoV-2, would have no knowledge of the radiotherapy administered to Mateo’s lungs just two years earlier. That fact is critically important. Regardless of whether he goes on to contract COVID again, or the severity of his symptoms, any analysis that is blind to his prior lung irradiation could yield misleading results. Suppose, for example, that Mateo does contract COVID a second time, through a variant, and again requires hospitalization for ARDS. Suppose, too, that pulmonary function tests, captured in EMR, suggest an even more severe case. This outcome may tell us as much about the long-term effects of lung irradiation as it does about the limitations of vaccination. Without claims data, researchers will miss that important confounder. Without EMR data, researchers may not realize severity of the outcome.
Looking forward in time, only claims data will tell us how diligent Mateo stays in refilling his prescription for his hypertension. If changes in blood pressure or lipid levels have any impact on his adherence, it will take the combination of EMR, which supplies the lab values, and pharmacy claims data, which supplies the fill dates, to understand that relationship. And what about the bone density loss Mateo’s PCP discovered? We can easily imagine Mateo suffering a hip fracture while traveling for work and receiving treatment at a hospital out of town. Any analysis relating the degree of bone loss to a later fracture needs data on both, but these facts will live in disparate EMR systems. Linking the data in both systems to Mateo’s single claim record ensures no independent or dependent variable gets missed.
Of course, Mateo is fictional. But he’s not entirely fictional. Every patient tells a story with chapters in different books. Before conducting your next analysis, consider what you gain by reading as many as you can!
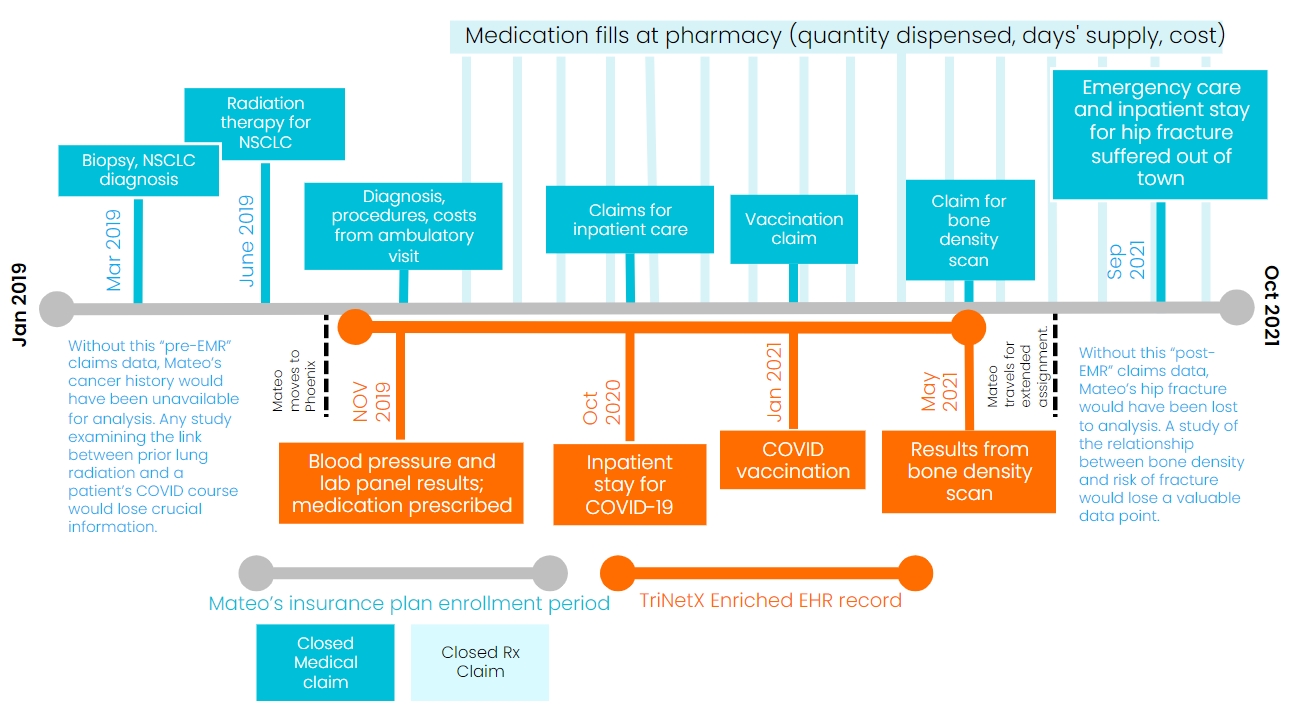